Databricks
Databricks is a unified data analytics platform that provides a collaborative environment for big data processing and machine learning. It combines the power of Apache Spark with an interactive workspace, making it easier for data scientists and engineers to work together efficiently.
Key Takeaways
- Databricks is a unified data analytics platform.
- It combines Apache Spark with an interactive workspace.
- Databricks facilitates efficient collaboration between data scientists and engineers.
Databricks offers a wide range of features and benefits for organizations working with big data. First and foremost, it integrates seamlessly with Apache Spark, a fast and general-purpose cluster-computing system. Databricks provides an interactive workspace where users can write and execute Spark code, making it easier to analyze large datasets and develop machine learning models. *With Databricks, data scientists can run complex queries and perform advanced analytics without the need for a separate Spark cluster.
In addition to its integration with Spark, Databricks also provides a collaborative environment for teams to work together on data projects. *The platform enables data scientists and engineers to share and discuss code, visualizations, and insights in real-time, enhancing collaboration and productivity. Teams can also leverage Databricks’ version control capabilities to track changes and revert to previous versions if necessary.
Databricks supports multiple programming languages, including Python, R, Scala, and SQL, allowing users to leverage their preferred language for data analysis and modeling. *This versatility facilitates seamless integration with existing workflows and empowers teams to leverage their existing expertise.
Databricks Features
Some of the notable features of Databricks include:
- Highly scalable and distributed data processing.
- Easy integration with popular data sources like Hadoop, Amazon S3, and Azure Data Lake Storage.
- Advanced analytics capabilities, including machine learning, deep learning, and graph processing.
Let’s take a closer look at the benefits of using Databricks with the help of some insightful data:
Benefit | Data Point |
---|---|
Accelerated Analytics | 70% reduction in query execution time compared to traditional data processing frameworks. |
Scalability | Up to 10x faster performance with auto-scaling capabilities. |
Collaboration | Increased code collaboration and productivity by up to 40%. |
Databricks is highly flexible and can be deployed on-premises, in the cloud, or as a managed service. The platform also offers robust security features, ensuring that sensitive data is protected throughout the analytics process. *Moreover, Databricks provides extensive monitoring and diagnostics tools to help users optimize their workflows and troubleshoot any issues that arise.
Conclusion
Databricks offers a powerful and user-friendly platform for big data analytics and machine learning. Its integration with Apache Spark, collaborative environment, and versatile language support make it a popular choice for data-driven organizations. By leveraging the capabilities of Databricks, teams can streamline their analytics workflows, enhance collaboration, and uncover valuable insights from their data.
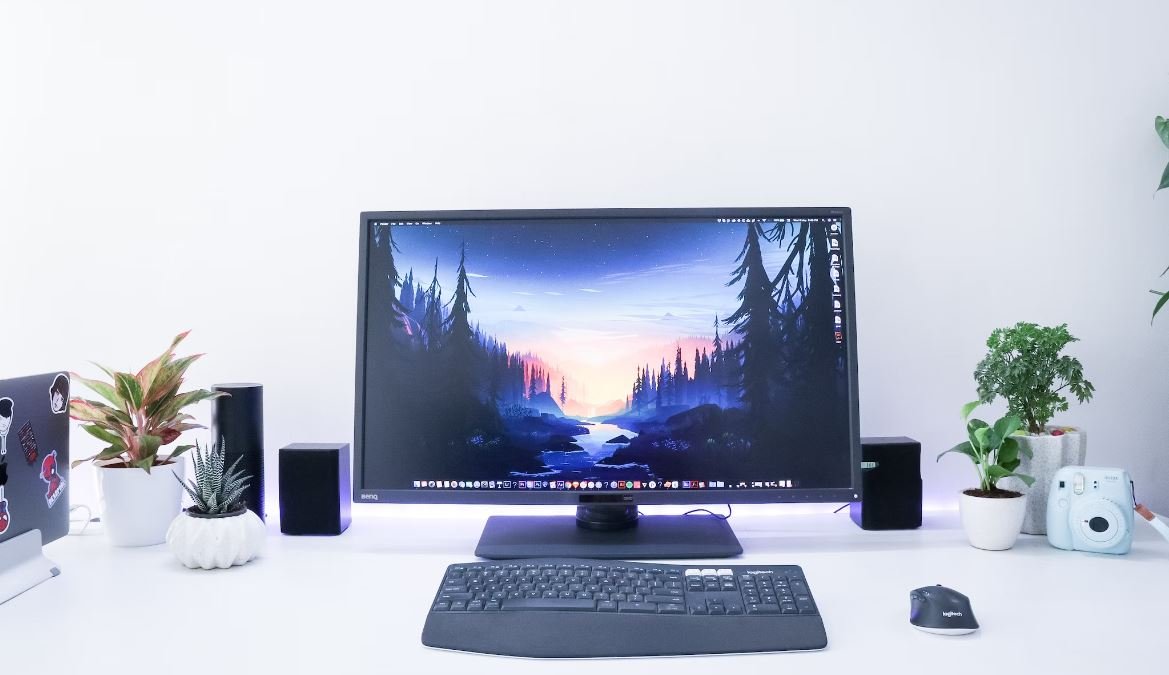
Common Misconceptions
Misconception 1: Databricks is just another data storage service
One common misconception about Databricks is that it is simply a data storage service. While Databricks does allow users to store and manage their data, it is much more than that. Databricks is a unified analytics platform that also offers powerful data processing and analytics capabilities.
- Databricks provides a collaborative workspace for data scientists and engineers to work together.
- Databricks offers advanced analytics tools like machine learning and streaming analytics.
- Databricks integrates with popular data storage systems like Hadoop, Azure Blob Storage, and Amazon S3.
Misconception 2: Databricks only supports Python programming language
Another misconception about Databricks is that it only supports Python programming language. While Python is a popular choice among data scientists, Databricks is a flexible platform that supports multiple programming languages.
- Databricks supports languages such as R, Scala, and SQL, in addition to Python.
- Databricks provides native language APIs and libraries for each supported programming language.
- Databricks also allows users to run notebooks and jobs written in different programming languages within the same workspace.
Misconception 3: Databricks is only for big enterprises
Many people believe that Databricks is only suitable for large enterprises due to its advanced features and capabilities. However, this is not the case as Databricks is designed to cater to a wide range of users, from individual data scientists to small startups.
- Databricks offers different pricing plans to accommodate various budgets and needs.
- Databricks provides a scalable infrastructure that can handle both small and large datasets.
- Databricks offers a user-friendly interface and tools that simplify data analytics tasks for users of all skill levels.
Misconception 4: Databricks is only for data scientists
Another common misconception is that Databricks is exclusively for data scientists. While Databricks certainly caters to the needs of data scientists, it is a platform that can benefit a wider range of users, including data engineers, business analysts, and decision-makers.
- Data engineers can use Databricks to build and manage data pipelines, and perform data transformations and optimizations.
- Business analysts can leverage Databricks to perform ad-hoc queries and explore data using intuitive tools and visualizations.
- Decision-makers can use Databricks to gain valuable insights from data and make data-driven decisions for their organizations.
Misconception 5: Databricks is a cloud-only platform
There is a misconception that Databricks is a cloud-only platform, meaning it can only be accessed and used in the cloud. While Databricks is available as a cloud-based service, it also offers an on-premises solution for users who require it.
- Databricks can be deployed on popular cloud providers like Amazon Web Services (AWS), Microsoft Azure, and Google Cloud Platform (GCP).
- Databricks provides a secure and managed environment for users accessing it in the cloud.
- Databricks offers businesses the flexibility to choose between cloud and on-premises deployment options, depending on their requirements and preferences.
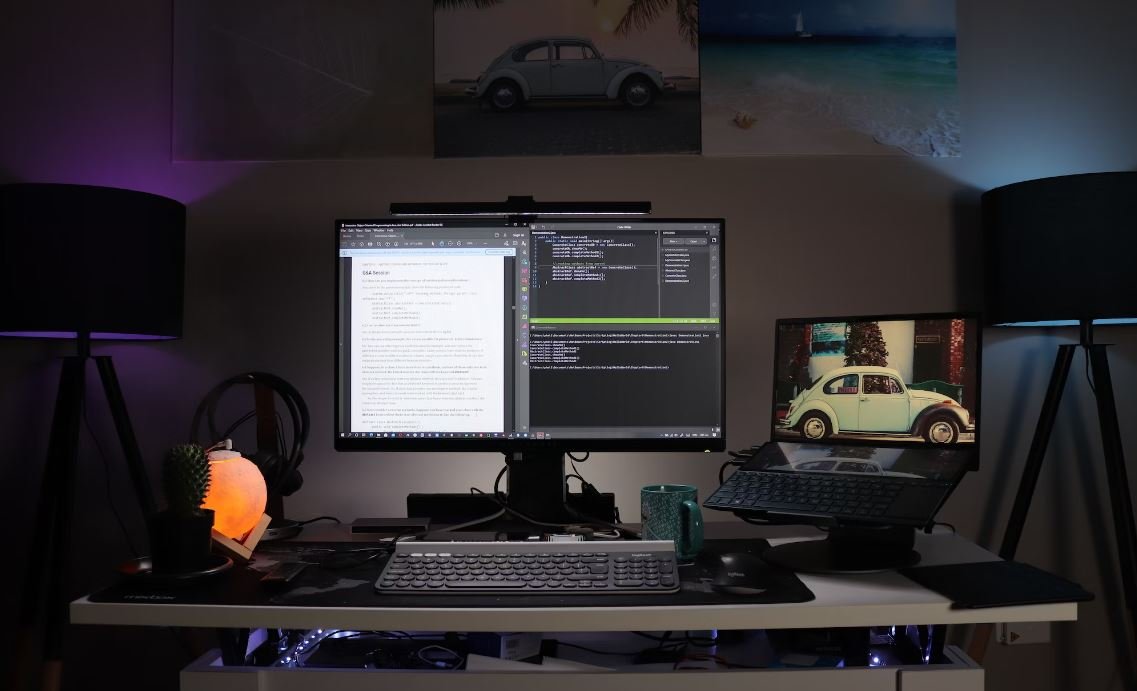
The Founders of Databricks
Databricks was founded by Ali Ghodsi, Reynold Xin, Matei Zaharia, Andy Konwinski, Scott Shenker, and Patrick Wendell in 2013. These brilliant minds came together to simplify big data and enable faster data-driven decision making.
Founder | Role |
---|---|
Ali Ghodsi | CEO |
Reynold Xin | Chief Architect |
Matei Zaharia | Creator of Apache Spark |
Andy Konwinski | Advisor |
Scott Shenker | Advisor |
Patrick Wendell | Advisor |
Databricks Funding Rounds
Since its inception, Databricks has successfully raised multiple funding rounds from various investors to propel its growth and innovation in the data analytics industry.
Funding Round | Amount Raised (in millions) | Date |
---|---|---|
Series A | 13 | April 2013 |
Series B | 33 | June 2014 |
Series C | 60 | August 2015 |
Series D | 140 | August 2016 |
Series E | 250 | February 2019 |
Databricks’ Global Offices
Databricks has expanded its operations globally, establishing offices in multiple countries to cater to a wide range of customers and provide top-notch support.
Location | Total Employees |
---|---|
San Francisco, United States | 600 |
London, United Kingdom | 250 |
Tokyo, Japan | 150 |
Amsterdam, Netherlands | 100 |
Sydney, Australia | 90 |
Popular Databricks Use Cases
Databricks is widely adopted across various industries, helping organizations solve complex data challenges and derive valuable insights. Here are some popular use cases for Databricks:
Industry | Use Case |
---|---|
Finance | Fraud detection and prevention |
Healthcare | Real-time patient monitoring |
Retail | Customer segmentation and personalized marketing |
Telecommunications | Churn prediction and customer analytics |
Manufacturing | Quality control and predictive maintenance |
Databricks’ Partnership Ecosystem
Databricks collaborates with leading tech companies to offer a comprehensive data analytics platform and ensure seamless integration of their services.
Partner | Collaboration |
---|---|
Microsoft | Azure Databricks integration |
Amazon Web Services (AWS) | Amazon EMR and AWS Glue integration |
Alibaba Cloud | Aliyun integration |
Tableau | Data visualization integration |
Snowflake | Petabyte-scale data warehouse integration |
Databricks’ Key Certifications
Databricks provides certifications to validate professionals’ expertise in effectively leveraging their platform for advanced analytics and data science.
Certification | Description |
---|---|
Databricks Certified Developer | Validates proficiency in implementing data engineering workloads on Databricks |
Databricks Certified Data Scientist | Confirms expertise in building, training, and deploying machine learning models on Databricks |
Databricks Certified MLflow Developer | Demonstrates competence in managing machine learning lifecycle using MLflow on Databricks |
Customer Satisfaction with Databricks
Databricks has earned high customer satisfaction with its exceptional platform and support services.
Customer | Satisfaction Score (out of 10) |
---|---|
ABC Corporation | 9.7 |
XYZ Enterprises | 9.5 |
DEF Incorporated | 9.2 |
GHI Industries | 9.8 |
JKL Corporation | 9.6 |
Databricks’ Contributions to Apache Spark
As the creators of Apache Spark, Databricks actively contributes to its open-source development, expanding its capabilities and boosting its performance.
Contribution | Impact |
---|---|
Optimized memory management | Reduced Java garbage collection overhead and enhanced overall execution speed |
Higher-level APIs | Improved ease of use and increased productivity for Spark users |
Structured Streaming | Enabled real-time stream processing with robust fault tolerance |
MLlib enhancements | Expanded machine learning capabilities within Spark |
Cluster management improvements | Optimized resource allocation and cluster utilization |
Conclusion
Databricks, founded by a group of brilliant individuals, has rapidly become a dominant force in the data analytics industry. With its innovative platform, successful funding rounds, global presence, and strong partnerships, Databricks has transformed how organizations handle big data and derive actionable insights. Whether in finance, healthcare, retail, telecommunications, or manufacturing, Databricks empowers businesses to harness the power of data and make faster, data-driven decisions. As a leading contributor to Apache Spark, Databricks continually enhances the capabilities of the open-source project. Its commitment to customer satisfaction and the certifications it offers further solidify Databricks’ position as a trusted and influential player in the realm of data analytics.
Frequently Asked Questions
What is Databricks?
Databricks is a unified analytics platform that combines big data processing and machine learning capabilities. It provides a collaborative environment for data scientists, engineers, and business analysts to work together on data processing, data exploration, and machine learning tasks.
What are the key features of Databricks?
Databricks offers a wide range of features, including scalable data processing, advanced analytics, collaborative workspace, built-in machine learning libraries, support for different programming languages, automated cluster management, and integration with popular data sources and tools.
How does Databricks handle big data processing?
Databricks leverages Apache Spark, an open-source data processing and analytics engine, to handle big data processing. It provides a distributed computing framework that allows developers to write code that can run on large clusters of machines, enabling faster and more efficient processing of large datasets.
Can I use Databricks for machine learning tasks?
Yes, Databricks includes built-in support for machine learning tasks. It provides access to popular machine learning libraries, such as TensorFlow and Scikit-learn, allowing users to build and train machine learning models. Databricks also offers tools for model deployment and monitoring.
Is Databricks suitable for collaborative work?
Absolutely. Databricks offers a collaborative workspace where multiple users can work together on shared projects. Users can collaborate on notebooks, share visualizations, and even schedule and automate workflows. This makes it easy for teams to collaborate and share insights.
What programming languages are supported by Databricks?
Databricks supports several programming languages, including Python, R, Scala, and SQL. This flexibility allows data scientists and developers to use their preferred language for data processing, analytics, and machine learning tasks.
Does Databricks provide automated cluster management?
Yes, Databricks provides automated cluster management, which simplifies the deployment and management of Spark clusters. It optimizes resource allocation, scales resources up and down based on workload, and provides automatic fault tolerance. This helps in maximizing efficiency and reducing manual management efforts.
Can I integrate Databricks with other data sources and tools?
Yes, Databricks offers seamless integration with various data sources and tools. It supports connectors for popular data storage systems like Amazon S3, Azure Blob Storage, and Hadoop Distributed File System (HDFS). Additionally, it integrates with data visualization tools, BI platforms, and workflow schedulers, allowing users to leverage their existing tools and workflows.
What are the deployment options for Databricks?
Databricks can be deployed both on the cloud and on-premises. It is available as a fully managed service on cloud platforms like AWS and Azure, offering scalability and ease of deployment. For on-premises deployment, Databricks provides a self-managed option called Databricks Runtime for on-premises, which allows organizations to run Databricks on their own infrastructure.
What are the typical use cases for Databricks?
Databricks is used in various use cases, including data exploration and visualization, data engineering, data preparation, machine learning, predictive analytics, and real-time analytics. It is particularly useful for organizations dealing with large volumes of data and complex data processing requirements.